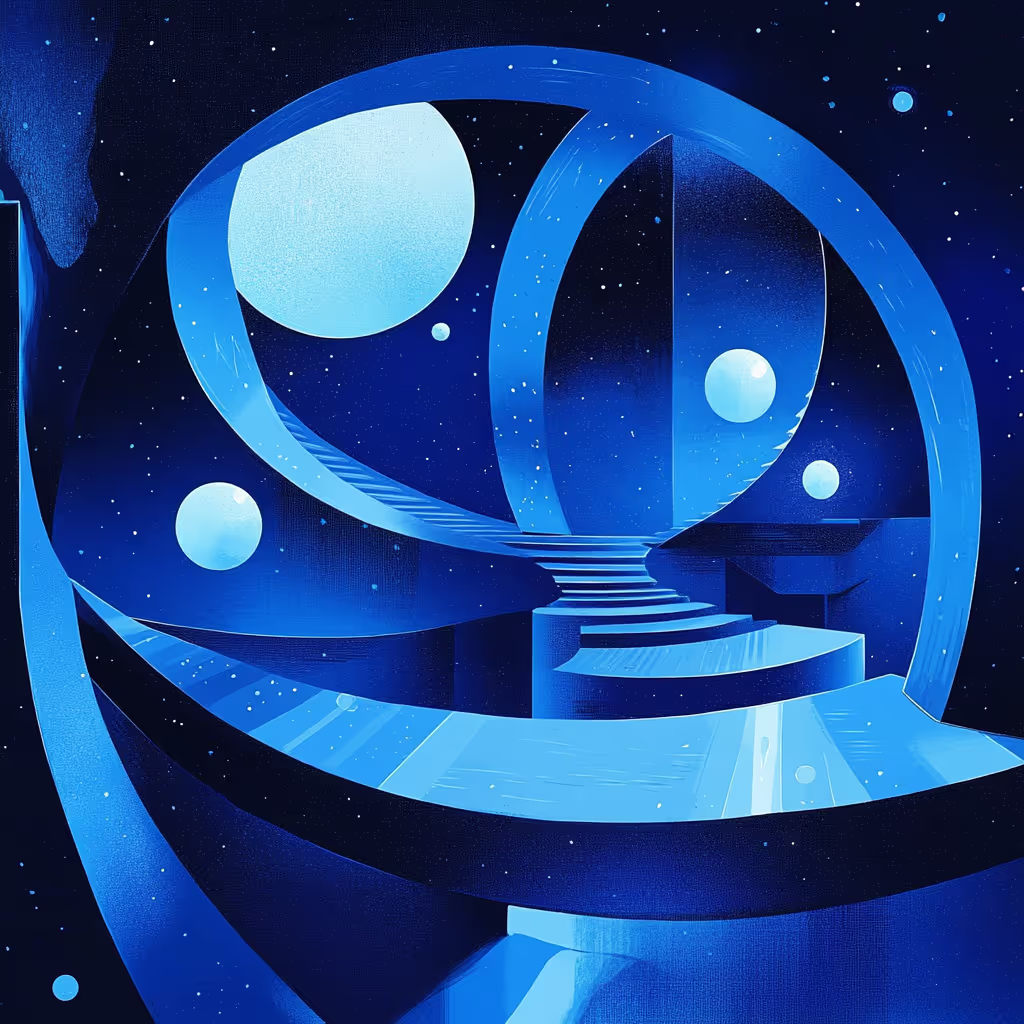
Transform Your Data Insights with the Best AI Tools for Data Analysts
Best AI Tools for Data Analysts in 2024
In 2024, innovative startups are redefining the best AI tools for data analysts with solutions that are more intuitive, automated, and powerful than ever. Here’s a selection of the top startups that are transforming how we analyze data.
Tool 1: Gravite.io (https://gravite.io)
Gravite.io is the rising star among data analysts. Why? Because it's not just another analytics tool; it's a smart companion that learns from your work habits and optimizes your data flows in a highly intuitive way.
- 5 killer features:
- Automation of repetitive tasks: Say goodbye to the tedious task of data cleaning; Gravite.io handles it intelligently for you.
- AI-based forecasting: With its machine learning algorithms, it predicts trends and helps you make data-driven decisions.
- Dynamic data visualization: Create interactive dashboards with just a few clicks, perfect for impressing during presentations.
- Real-time collaboration: Share your analysis and collaborate seamlessly with colleagues directly on the platform.
- Advanced customization: The tool adapts to your work style and preferences, offering a unique user experience.

Tool 2: DataRobot (https://www.datarobot.com)
DataRobot positions itself as another leader among the best AI tools for data analysts. It's perfect for those who want to automate predictive model building without any hassle.
- 5 killer features:
- Automated modeling: Save time with the automatic creation of machine learning models.
- Quick model evaluation: Get performance scores at a glance to select the best model.
- Seamless integration with BI tools: Connect DataRobot directly with your favorite Business Intelligence tools for smooth analysis.
- Support for multiple languages: Whether you prefer Python, R, or even SQL, this tool has you covered.
- AI-augmented data exploration: Quickly identify hidden insights with augmented analytics.
Tool 3: Tableau (https://www.tableau.com)
Tableau remains a standout among the best AI tools for data analysts, especially for those who love data visualization.
- 5 killer features:
- Intuitive data visualization: Easily transform your data into impressive charts and graphs.
- Integration with various data sources: Connect to a wide range of data sources effortlessly.
- Simplified predictive analytics: Use predictive models without needing a PhD in statistics.
- Strong community support and abundant resources: Access an active community and tons of learning resources.
- Interactive dashboards: Create dashboards that your colleagues will love to explore.
Tool 4: Alteryx (https://www.alteryx.com)
Alteryx is another key player, particularly appreciated for its automation and predictive analytics capabilities.
- 5 killer features:
- Automated data workflows: Create automated workflows to save time and reduce errors.
- Self-service data preparation: Prepare your data without needing help from a data engineer.
- Integrated geospatial analysis: Add a geospatial dimension to your analyses effortlessly.
- Advanced predictive modeling features: Develop robust predictive models with an intuitive user interface.
- Enhanced team collaboration: Easily collaborate with different departments in your organization.
Tool 5: RapidMiner (https://rapidminer.com)
RapidMiner is a favorite for data scientists who like to have visual control over the entire analysis process.
- 5 killer features:
- Visual data science platform: Design your analysis models visually, without complex code.
- Extensive library of predictive models: Use a variety of predictive models to refine your analyses.
- Feature engineering capabilities: Improve the quality of your models with feature engineering tools.
- Simplified model deployment: Move from modeling to deployment in a snap.
- Integration with various data environments: Compatible with a wide range of data environments.
Tool 6 to 10:
- H2O.ai (https://www.h2o.ai)**
- Open-source AI models: Benefit from the latest open-source innovations in AI.
- Multi-language support: Use the programming languages you prefer.
- Scalable machine learning algorithms: Scale up with algorithms optimized for big data.
- Automated Machine Learning (AutoML): Save time with AutoML.
- Visualization of learning results: Understand your model results with clear visualizations.
- BigML (https://bigml.com)
- User-friendly machine learning platform: Perfect for those new to machine learning.
- Automated predictive modeling: Get predictions in just a few clicks.
- Robust API for developers: Easily integrate BigML into your applications.
- Supports online learning: Update your models with new data in real-time.
- Model interpretability features: Better understand how and why your models make predictions.
- Knime (https://www.knime.com)
- Visual analytics platform: A favorite for those who like to visualize their workflows.
- Easy integration with Python and R: Ideal for mixed data science teams.
- Wide range of data science modules: Access a broad range of tools for data analysis.
- Support for explainable AI: Ensure your models are transparent and explainable.
- Rich ecosystem of plugins and extensions: Customize Knime to your liking with numerous plugins.
- Sisense (https://www.sisense.com)
- Advanced analytics dashboards: Create stunning data visualizations.
- Augmented analytics features: Use AI to gain insights you might not have noticed otherwise.
- Real-time data visualization: Analyze your data in real-time for quicker decisions.
- Integration of AI and ML for forecasting: Combine AI and ML for robust predictive analytics.
- User-friendly interface: A design that makes it easy for everyone to use.
- Databricks (https://databricks.com)
- Unified platform for big data and ML: An all-in-one solution for big data and machine learning.
- Collaborative with shared notebooks: Perfect for real-time collaboration with remote teams.
- Optimized for Apache Spark: Leverage the power of Spark for ultra-fast analytics.
- Multi-cloud support: Works across multiple clouds, perfect for modern enterprises.
- Automated data pipeline management: Simplify data management with automated pipelines.
Future Trends in AI Tools for Data Analysts
Now, let's look to the future! Here are the trends to watch for the best AI tools for data analysts:
- Capturing all qualitative data (speech to text): AI tools are becoming increasingly effective at converting unstructured data, like speech or long texts, into actionable data. Imagine a tool that listens to your meetings and automatically extracts key points and relevant insights!
- Data-driven decision-making: Data is no longer just for post-event analysis; it is now a key player in real-time decision-making. AI tools will increasingly integrate simulation capabilities, allowing you to see the potential impact of each decision before you even make it.
- Employees focusing on high-value tasks thanks to AI: With the automation of repetitive tasks, data analysts will be able to focus on more complex analyses, strategy, and decision-making, maximizing their impact within the company.
- Examples of what AI could be tomorrow:
- Real-time data assistant: An AI assistant that guides you and suggests analyses to perform based on the data you're handling.
- Advanced explainable AI: Models capable of transparently explaining their decisions and predictions, making them easier to adopt by non-technical users.
- Hyper-contextual personalization: Tools that adapt not only to your preferences but also to the specific context of each project or analysis.
- AI-augmented collaboration: Tools that facilitate cross-functional collaboration by understanding and translating the needs of each team.
- Proactive data quality monitoring: Tools that automatically detect and correct data errors before they affect analysis.
There you have it! With these startups and trends, you'll have a significant edge in 2024. They mark the beginning of a revolution in how we approach data analysis, making the process more intuitive, automated, and collaborative. Ready to dive into the future of data analysis? Let's go!